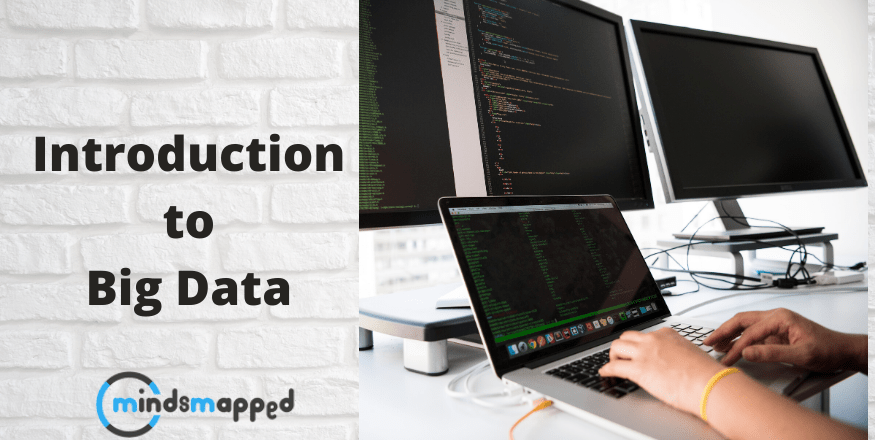
What is Big Data? Simple Explanation with E-Commerce Examples
Introduction to Big Data?
This age unlike any is confronted with growth from the size of information generated and captured. Data growth has undergone a renaissance, influenced by the ubiquity of the net and cheaper processing power of computers. This has resulted in a paradigm change in the e-commerce industry; as information is no longer regarded as the byproduct of the business activities but as their main advantage, supplying key insights into the demands of their clients, forecasting trends in customer’s behavior, democratizing of advertisement to suits customers varied taste, in addition to providing a performance metric to evaluate the efficacy of meeting clients’ needs. Today, a greater portion of economic activities takes place electronically as individuals and organizations participate in buying and selling through the web and exchange of information. E-commerce vendors have taken advantage of the internet’s use to enhance brand awareness, and increase revenue.
The development of data analytics facilitated data-driven and informed strategies as businesses analyze the data trail left behind by buyers and would-be buyers to obtain a better understanding of consumer behavior. The opportunity of obtaining such unique insight makes it possible for businesses to improve customer service, make a smart business move, and provide democratized services and products to its customer base.
Currently, there is no unified definition for the term”Big Data”, but the most commonly accepted definition of big data is in relation to 3 attributes, volume, velocity, and variety also called 3 V’s.
Variety refers to the hierarchical character (composed of structured and unstructured datasets), Velocity depicts the rate at which data is recorded, and Volume denotes the size of information (usually in Petabytes, Exabyte and Terabytes). Because of these characteristics, it is impossible to manage and analyze big data using conventional databases. However, using specific tools and technology (for example, Hadoop Distributed files system), big data can be efficiently managed (management procedures of such enormous data includes storage, processing, real-time and near-real-time analysis). Additionally, when particular data mining algorithms (for example, machine learning and clustering algorithm) are introduced into the big data analytical framework, enterprises are able to derive insight from the data.
In the year 2012, research carried out on businesses in the United Kingdom reveals that 23% uses social media platforms to react to customer opinions and questions. This poll evidences the fact that the exchange of digital information via social networking sites has favorably impacted e-commerce in the areas of revenue and brand awareness. With the application of data analytics to the enormous data generated there’ll be more educated strategies for companies. Such that e-commerce vendors and businesses can analyze and harness the insight derived from the enormous data that has been generated from Electronic Data Interchange (EDI), so as to obtain a better understanding of customer behavior.
For the purpose of this article, let us restrict the operations of data analytics into three classes as follows:
1. Social Media Analytics: Refers to the analysis of a large volume of data generated from social networking applications and sites
2. Predictive Analytics: Refers to the use of data to forecast consumer tendencies and behaviors.
3. Mobile Analytics: This refers to the analysis of large volumes of data generated from gadgets, tablet computers, and cellular phones.
Social Networking Analytics (SMA)
The advent of social networking technology has changed commerce forever. This entails the collection of data from social networking sites/applications (for example, wiki, Facebook, Twitter, GooglePlus, blogs, etc) and assessing such data to gain insights/knowledge. As it bears the 3V characteristics, social networking data can be categorized as big data. (For example each day there’s about 35 million status upgrades and more than 100,000 tweets per second on Twitter).
Social Networking websites are not just networked of interconnected relations, but it’s a virtual society where the members interact, exchange information, and develop relationships. These activities are capable of tilting a consumer’s opinion on a particular brand. These findings are based on a survey carried out on 6,000 social media users, it was shown that:
- Nearly 40% of social networking users have purchased a product after”favoriting” or sharing it on a social networking site.
- 71% of social media users are more likely to buy based on referrals.
- 74% of customers depend on social networking networks when making decisions about what to buy.
- Facebook affects 30.8% of social networking users’ buying habits, while LinkedIn and YouTube affect 27% of social networking website users respectively.
Because of the statistics, e-commerce vendors are adopting social networking analytics for the following reasons:
- To gain competitive advantage
- Drive customer traffic
- Boost customer loyalty and retention,
- Improve sales and revenues, improves customer satisfaction; and most importantly to create brand awareness and build a reputation.
Walmart (Known as ASDA in the United Kingdom) is an American multinational retail corporation. In April 2011, Walmart bought Kosmix, a social networking startup focused on e-commerce. Kosmix developed a software program that had the capacity to search and examine social networking applications (such as Twitter, Facebook, Blog spots) in real-time so as to offer personalized insights into users. Kosmix was also creating a knowledge base application known as the”Social Genome” that had the capability to capture information and connections about people, events, topics, products, places, and associations. When Walmart bought the firm they changed the title to @WalmartLabs. Walmart’s Social Genome (publicly called Walmart Shopycat) applications constantly capture data from social networking sites in real-time with countless entities and relationships. The program performs a semantic analysis of social networking and feeds its output to custom-built e-commerce programs for Walmart. In January 2013, the business reported that its social networking analytics applications project is capable of indexing and searching 60 billion social media documents and subsequently assist its marketers to understand sentiments, trends, and popular products on a real-time basis. The program also has the capability to observe sentiments based on geographical locations and forecast trends in all Wal-Mart shops in addition to e-commerce shops.
Predictive Analytics
Predictive analytics makes it possible for organizations to respond proactively to business challenges. This involves using past/historical data to predict future trends. This analysis uses machine learning algorithms and models to learn from data and to identify patterns. Using the big data analytics platform to analyze these data (alongside data mining and machine learning algorithm), e-commerce sellers can efficiently predict consumer behavior quicker, more effectively, and at greater effective cost.
Large e-commerce sites usually have thousands of products and services available for sale. Searching and navigating for a product or service out of thousands on the website is stressful and time-consuming for consumers. However, with the availability of recommender systems, e-commerce sites and applications can quickly identify and predict products and services that closely suit what customers need. Amazon.com is the world’s largest retail store. Amazon started off as an internet book store and quickly diversified into manufacturing and sales of consumer products, electronic equipment, and household items amongst others. At Amazon, product recommenders are utilized to personalize each shopper’s experience. Products are adapted to suit every shopper’s preferences (on a real-time basis). This is one of the incomparable advantages as well as the challenge of big data as Amazon captures millions of shopper’s data to gain this edge. Amazon uses clustering algorithms and collaborative filtering to group shoppers based on preferences. Their product recommender system groups shoppers into clusters/groups based on:
• Similar search
• Item to Item collaborative filtering.
Search based (also called content-based search) uses a customer’s buying history and rated products and services to create a search query that locates other things close to customers’ preferences. For example: if a customer purchases a DVD called the “Titanic”. The product recommender will urge movies either from similar actors, similar genres, or similar directors.
Mobile Analytics
There has been a growth in the amount of cell phone users. In like fashion, there is a radical shift in the applications of mobile phones, formerly cellular phones were only used as a mere tool for communicating. However, in this age, mobile phones are utilized to perform an assortment of functionalities such as send emails, games, track individuals (using GPS), perform banking transactions, online shopping among others, Research has shown that more people now use their phones for online shopping. Consequently, mobile phones are used as an integral tool in e-commerce platforms. By leveraging the enormous data generated from mobile phone data exchange, e-commerce companies can provide value-added services such as geo advertising and location-based discounts. E-commerce businesses can also identify location patterns of customers using existing customers’ data to communicate customized messages to customer prospects in a timely manner.
Mobile phone analytics is gaining more ground in e-commerce because it is cheaper, effective, and serves as a timely platform to promote products and services. It makes it easy for advertisements to be communicated to customers in real-time based on their locations, and proximity to a store, by taking advantage of data mined from GPS data of customers’ mobile phones.
Mobile phone analytics and location-based services are aimed at supplying personalized mobile transactions to a particular customer at a specific place, by using awareness of their present location. These strategies have assisted e-commerce vendors in repositioning themselves and their businesses to proactively respond to the challenges and opportunities of the e-commerce environment. The technologies that enable location-based marketing to thrive include the use of RFID tags, Bluetooth, and GPS to identify users’ proximity to a particular location. Bluetooth location-based advertisement uses Bluetooth to target users for special and discounted sales at a specific period of time. For example, customers of a particular e-commerce store can be targeted for discount sales, with such discount offers being communicated to the customers using Bluetooth location-based systems to target such customers when they are close to the e-commerce store. To start a brand new product called the”Adizero F50 soccer cleat” Adidas partnered with Mullen’s media hub (Mullen is a media and advertising company), the significant target for this effort was mobile users across multiple mobile platforms (Android, Windows, iOS). The aim was to invite them to Adidas’ light series, which introduced the Adidas new merchandise. Using location-based targeting, the business was able to target users inside a three-mile radius of Penn Station and push mobile advertisements in related mobile programs (such as Facebook) and sometimes SMS. The ad read: “Adidas and Messi — After Dark Tonight.” Users were redirected to a landing page that displayed the promotional video explaining the event, the place, and the time. This accomplishment was made possible using big data analytics to filter customer’s locations and streamlining (in real-time) to convey advertising notifications to targeted users. The results of this effort was a massive success. It reached 7.3 million consumers, generated 45,856 clicks into the Adidas store, generated over 15,000 shares on social networking sites, and 7.6 million people saw it live.
Final Words – Why Big Data?
Though big data analytics hold a huge potential in generating revenues and increasing profits by providing business insight and analyzing customers’ behavioral patterns, it has also come with some downsides. Research has shown that the largest obstacles to big data analytics are training and privacy concerns. Most of the customers are concerned about how their personally identifiable information is used. Organizations in possession of customers’ digital identity and footprint are at liberty to use it for any intent. Unfortunately, some of these organizations have abused this liberty by immorally exploiting customers’ data. Consequently, privacy experts have concluded that big data analytics is an infringement on the privacy of our lives.
Despite these challenges, many organizations are currently moving forward to adopt big data as a significant part of their strategy. The majority of the top business organizations in the USA are able to attain these feats by exploiting big data analytics. Noting the opportunities that big data analytics fosters for the e-commerce enterprise, there is a need for more personnel training and governance framework that will put structures in place that guard against the malicious and immoral use of customers’ data.