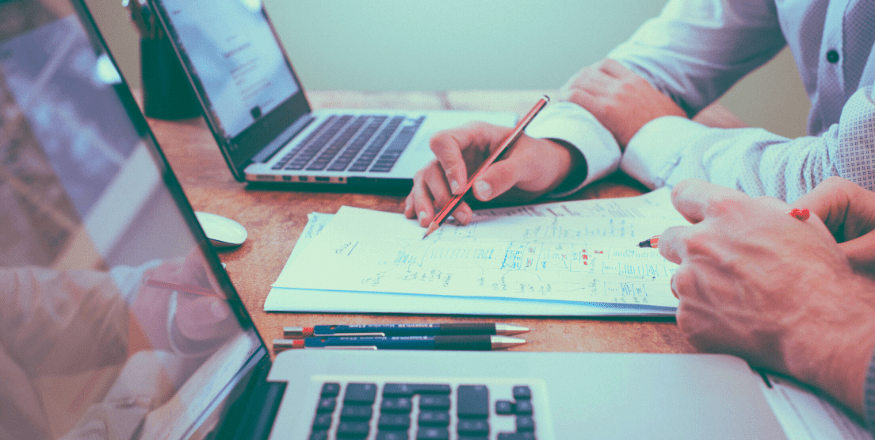
Introduction to Big Data. Simple Explanation with an Insurance Use Case
Application of Big Data in the Insurance Industry
The big data era has generated a new tide of innovation in the insurance industry. Access to a rich variety of data sets, alongside the ability to analyze the data sets, has enabled innovations and insights that were formerly impossible. This has opened the door to the era of dynamic risk management and refines the practices of modeling catastrophe risk.
Dynamic Risk Management
Consider the automobile insurance sector, the insurance industry commonly refers to this type of insurance as Usage-Based Insurance. However, the application of dynamic risk management goes far beyond the scope of driving and automobile insurance. Dynamic risk management is an advanced version of actuarial science. Actuarial science deals with the collection of relevant data, using algorithms and models to factor risk, and then forming a conclusion. Dynamic risk management involves real-time analytics based on a stream of data. Let’s examine the two models with an example of car insurance for a twenty-year-old woman:
Actuarial Insurance Model
Collect all the data available for the twenty-year-old — her driving record, type of vehicle, location, criminal record, etc. Combine these data with demographic data for her gender, age, location, and employment record. Leverage the concepts of probability, mortality, demographics, and compound interest to estimate the risk and reward. Then, recommend a policy to the woman, based on these factors.
Dynamic Risk Management Model
Fix a sensor in her car and let her go about her normal life while the sensor monitors information like mileage, driving periods, speed of the vehicle, acceleration, and deceleration. This on-board monitor is constantly pricing her insurance policy based on her driving behavior. If she is a careful driver, her next premium will be lower. The policy is customized for her and is based on real-time data analytics, as opposed to estimates.
There is now increased acceptance for dynamic risk management. In March 2011, the European Court of Justice maintained that taking the gender of the insured individual into consideration as a risk factor in insurance contracts constitutes discrimination. From December 2012, insurers doing business in Europe no longer charge different premiums on the basis of the insured person’s gender. The insurance industry has good reasons for using gender as a means of quantifying risk. Men under the age of thirty are twice likely to be involved in a car accident as their female counterparts. Insurers also have empirical evidence to prove that the claims they receive for young men are more than three times as large as those for women. Debates revolving around gender equality have rightly established that it is inconsiderate to discriminate against young men. Furthermore, these debates have underscored the need for more detailed metrics for predicting risk rather than the undiplomatic use of gender. The response to this discriminatory policy requires better models and dynamic risk management based on the real-time driving know-how of the individual.
The application of dynamic risk management encompasses any data-centric insurance operation; for instance, It is readily employed by insurance organizations that leverage telematics or data points about a consumer in a lending scenario. Dynamic risk management is gradually becoming a routine due to the expanding influence of big data.
The insurance industry can make themselves more reputable by using dynamic risk management as a means for encouraging a change towards good behavior. The use of big data analytics to investigate individual risks will motivate the client to behave in a more responsible manner. The insurance industry gives notification of difference in cost incurred from careless and careful lifestyle. The noticeable difference can spur clients toward a low-risk lifestyle.
Big data is can create revolutionary risk models that are extremely accurate, capable of being updated in real-time, and centers on the individual client. This is an anathema to those hoping to exploit insurance as a reason to indulge in excessive risk-taking, but it is a commendable innovation to those that will like to be rewarded for managing risk more responsibly. As more people opt for dynamic risk management, there will be lesser automobile accidents, our roads will be safer, and healthcare bills will be reduced to the barest minimum.
Catastrophe Risk
Catastrophe risk models are computer-based methods of measuring potential risks because of natural catastrophes such as earthquakes, windstorms, and floods. In the 1980s, advancements in information technology and geographic information systems generate estimates of catastrophe risks by overlaying the properties in an insurer’s portfolio with the potential natural disaster sources in the geographic area. After Hurricane Andrew made landfall in 1992, causing losses of $15.5 billion, the insurance industry responded by embracing catastrophe risk modeling as a new philosophy for doing business. The quick acquisition of catastrophe models was also necessitated because one of the original vendors, AIR Worldwide, accurately predicted in real-time the losses from Hurricane Andrew could exceed $13 billion. Catastrophe risk-management models analyze a variety of potential risks that appear in a geographic location. Geography is key, as certain types of risk are inherent in certain locations.
The three major catastrophe risk-modeling vendors are RMS, AIR Worldwide, and EQECAT. The central responsibility of insurance is about pricing uncertainty; that is the obligation of these three vendors. They predicted a better pricing policy will be achieved, as models get smarter by analyzing big data, to gain new insight
Open Access Modeling
Inspired by all the remarkable achievements of catastrophe modeling, more improvements are still very much needed. The sudden and colossal devastation of the floods in Thailand in 2011 was not properly modeled when a huge loss of about $45 billion was incurred due to disruptions to manufacturing logistics. This domino effect of natural disasters and economic disruption is very difficult to model. Modeling predicts risk. Data refine models. The use of big data will reduce uncertainty in models, and improve its pricing mechanism. This will invariably increase the accuracy of the model in predicting risk.
Risk assessment is a forecasting process, and it is an established fact in the discipline of forecasting that seeking the opinion of multiple forecasters before taking a decision is a clever step. From a modeling viewpoint, multiple opinions equate to using a collection of diverse models, also called ensemble forecasting techniques, are now readily employed by many weather service providers. Sadly, catastrophe-modeling vendors are not willing to share their commercial models, often referred to as “black boxes” with other vendors in the industry. This secretive mode of interaction among the vendors has prevented cross-fertilization of ideas and expertise capable of hurling the industry to a new height. The consequence is the inability to create an ensemble of sophisticated models, which is against the spirit of scientific and technological advancement. Although different stakeholders implemented policies to suppress this practice, more effort is still required to attain a state of total open source in this domain.
The Global Earthquake Model (GEM) was formed in 2009 by the Organisation for Economic Co-operation and Development (OECD) in the realization of the necessity for catastrophe modeling to be made available to everybody free of charge. The GEM encourages an open-source stance to research, data collection, and modeling. The GEM was set up to emphasize the need for more transparency in estimating the risks connected with natural disasters. In 2014, a consortium of 21 stakeholders unveiled the Oasis Loss Modeling Framework, a framework for independent catastrophe modeling. The consortium claimed that the Oasis Loss Modeling Framework is the most significant development in the modeling of natural catastrophe losses for two decades. It is an open-source approach to making software, data sets, and methodologies, freely available for use by any party that is interested. It operates as an open market, for models and data. Anyone can get assistance, anyone can offer assistance.
Opportunities
The total losses from catastrophes were a whopping $192 billion in 2013. When dealing with risks at this frightening scale, the idea of using big data to boost model efficacy, reduce risk, and become more competitive becomes more appealing. The following is a set of challenges and opportunities that the insurance industry is grappling with when incorporating big data:
Computation
Big data are enormous and high dimensional and these come with computational challenges like scalability, noise accumulation, and storage bottleneck.
Interpretation
It is important to measure uncertainty in the data and employ sentiment analysis techniques to uncover trends in unstructured data. Collaboration with third parties, introduces a unique perspective, leading to a more enriched interpretation.
Transparency
A gap exists between quantitative analysis (data and models), and how the quantitative outcomes are implemented by policymakers. For outcomes to be useful, this gap has to be closed or narrowed.
Prediction
Evidence-based policies come with their own regulations and protocols. Varying levels of confidence in recommendations should be clearly spelled out.
Reputation risk
Unfavorable working conditions, as well as lax tax laws and perceived evasion, makes it an uphill task to have access to relevant data necessary for building efficient models capable of providing an objective assessment of risks.
Scenarios
Processes, conclusions, and recommendations, based on probability, will increase competitiveness, make models more efficient, and reduce risk. The occurrence of diverse catastrophic risks in the world continues to evolve, elevating business challenges, and opportunities. With globalization and increased connectivity through conversations via virtual communication software, video conferencing, and social media websites and apps, comes new risks, that poss a great threat to civilization and our collective existence.
Local versus global
Deploying local models to calibrate potential global impacts have proven to be unsatisfactory, often leading to more risk than perceived.
So, how does big data fit into this picture? Let us start by examining the timeless equation for risk:
Risk = Hazard × Exposure × Vulnerability
In building a catastrophe modeling from the equation, the risk is taken as the expected financial loss measured in dollars, the hazard is the peril under consideration (e.g. storm, hurricane, flood or earthquake), exposure gives information about the geographical locations of assets, and vulnerability measures the extent of the damage. This model equation incorporates all the emerging risks, challenges, and opportunities of controlling these risks. However, the model is only as accurate as the data that is fed into the equation. Using this equation strengthens quantitative predictive modeling by augmenting it with qualitative judgment. Big data techniques will support the union of scientific knowledge and empirical analysis. The aim is to use the equation as a game-changer to provide predictive analysis of risk, as against retrospective analysis of risk. This is what distinguishes an insight from history.
A method for dealing with time-varying risks due to climate change is a brilliant example of prospective risk modeling. Historical hurricane records reveal the past, but the future may present something entirely different. Dynamical atmospheric models, similar to climate and numerical weather prediction models are now used for producing synthetic hurricanes as outputs. By producing different climate scenarios, it is possible to generate a synthetic list of hurricanes that can then be used to assess the potential losses. Researchers in Earth and Environmental Sciences have succeeded in developing a procedure for using data generated by atmospheric models to provide enough long-term records to quantify windstorm risk to infrastructure, such as wind farms, where only short-term wind-speed records are available.
Big data has transformed insurance as a result of the many different sources of information that can be utilized to make risk models better. Satellite imagery is used to determine the exact location and size of physical assets. Digital connectivity such as virtual communication and social media provide a platform for allowing people to help with the collection of data necessary for quantifying risks.
In most cases, it is the vulnerability component of the risk equation that is usually lacking. Data about the structure of houses, for example, must be obtained from non-conventional sources. This form of collective data gathering, referred to as crowd-sourcing, is very efficient in developing countries where risk models are absent.
Final Thoughts
As expected, resistance to change was a major constraint found everywhere within the large insurance organizations. Business methodologies have been forged with time and habitual practices, and the desire to change has been stifled. However, readiness to change and awareness that change is required are two different perceptions. The industry knew the modus operandi needs to change but the stakeholders are not willing to step out of their comfort zones and break new ground.
The role of actuaries is changing. As they become more data-centric, the challenge for actuaries will be to find new ways to manage data. Taking a large mass of data that is high dimensional and converting it into a simpler structure that the unsophisticated mind can understand is not an easy task. Large and complex data sets require a proper set of tools to assist the actuary in his evolving role. However, it is not only about proper tools, but it is also about a cultural shift. The actuary and the industry must be prepared to evolve or lead the way to chart a new path for the industry if they are going to surmount emerging challenges.